Pricing GenAI – A review of existing models and a look toward the future
Author
James Wilton
Managing Partner
Pricing GenAI – A review of existing models and a look toward the future
AI has changed everything—from how we work to how we interact with technology. But for companies with AI products and features, there’s one critical question that still has no clear answer: How should it be priced?
It’s well-documented that GenAI introduces fundamental challenges to traditional SaaS pricing models. The cost structures are different, usage can be unpredictable, and the way AI delivers value doesn’t always align with common SaaS pricing frameworks.
The market is clearly still figuring this out. Some companies are taking usage-based approaches (OpenAI), while others lean on per-seat pricing (Copilot), outcome-based pricing (Zendesk), or hybrid models (Jasper). Each approach comes with its own benefits and pitfalls.
So, what’s the right way to price AI? It depends.
We’ll break down the key pricing models for AI, the trade-offs they create, and where the industry could be heading.
The Core Challenge: AI’s Cost & Value Are Hard to Align
Traditional SaaS pricing models—like per-seat or feature-based pricing—work well because they scale predictably with customer adoption. AI breaks this pattern in three ways:
1. Compute Costs Are High and Variable
– Unlike traditional SaaS, where marginal costs are low, AI models are expensive to run. Every query, every generated response, and every API call burns significant compute power.
2.
Usage Doesn’t Always Equal Value
– AI products are often priced based on API calls, tokens, or queries—but that doesn’t that truly capture the value the AI provides. More usage doesn’t always equate to more value for the customer.
3. Customers Want Predictability – Businesses are used to – and expect - predictable SaaS pricing. But AI pricing can be volatile, making budgeting difficult. This leads many customers to push back on pure usage-based pricing models.
Given these challenges, AI companies are experimenting with different approaches to pricing.
The Most Common AI Pricing Models (And Their Trade-Offs)
There’s no
one-size-fits-all pricing model for AI, but here are the most common approaches vendors are using today:
1.
Per-Seat Pricing (Familiar, but Misaligned with AI Costs)
This is the
default SaaS model—charging per user per month. It’s easy for customers to understand and provides
predictable recurring revenue.
✅
Works well when each user gets distinct value from the AI.
❌
Problem: AI’s
costs don’t scale per user—they scale with compute and usage.
📌
Example: Microsoft Copilot – Copilot is priced at
$30 per user per month. Microsoft likely chose this because it’s easy for enterprises to budget and aligns with
how they already buy software.
💡
The challenge? Not every employee needs AI, so companies will hesitate to roll it out broadly at that price point.
2. Usage-Based Pricing (Cost-Aligned, but Unpredictable for Customers)
Since AI’s costs scale with usage, many vendors are adopting
pay-as-you-go models where customers are billed per:
- API call
- Token processed
- AI-generated output
✅ Works well when AI usage clearly correlates with business value.
❌ Problem: Customers hate unpredictable costs. AI usage can be hard to forecast, making budgeting a nightmare.
📌 Example: OpenAI API – OpenAI charges per 1,000 tokens, aligning pricing with its direct compute costs.
💡 The challenge? Many businesses hesitate to adopt products where they don’t know their monthly bill in advance.
3.
Outcome-Based Pricing (In Theory, the Gold Standard—But Hard to Execute)
What if AI vendors only got paid when their AI actually delivers results?
This sounds great in theory—customers love the idea of paying only for value. But in practice, it’s difficult to execute because:
- Defining an “outcome” is tricky. What exactly counts as success?
- Attribution is a nightmare. If sales increase, was it because of AI, better sales reps, or a strong market?
- Revenue gets delayed. Vendors may have to wait months before getting paid.
📌 Example: Conversational AI & Zendesk – Some conversational AI vendors have experimented with pricing based on the percentage of customer inquiries handled by AI rather than humans.
💡 The challenge? Most customers still prefer flat pricing because they value budget predictability over outcome-based models.
4. Hybrid Pricing Models (Where Most of the Industry Is Heading)
Given the downsides of the previous models, many AI companies are now adopting hybrid approaches that blend different models.
Some common hybrids:
✔ Per-Seat + Usage Tiers – A base subscription + pay-as-you-go for additional usage.
✔ Usage-Tiered Per-User Pricing – Instead of charging a flat $30/user, charge per user with limits on AI usage (e.g., X queries per user).
✔ Base Subscription + Performance Bonuses – Charge a subscription fee but include an outcome-based component for added revenue.
📌 Example: Jasper AI – Jasper offers tiered subscriptions with AI word limits. Customers pay a base rate, but higher usage unlocks more capacity.
Where AI Pricing Is Headed Next
The industry is still experimenting, but a few trends are emerging:
🔹
More Structured Hybrid Models – The best AI pricing strategies will likely mix subscription fees, usage-based pricing, and value-based elements.
🔹
Greater Customer Control Over Usage – Customers don’t want surprise bills. Expect more pre-purchased credits, usage caps, and commit-based discounts.
🔹
New Metrics for Pricing AI Fairly – The industry needs better ways to align pricing with real business value rather than just raw consumption.
The AI landscape is continuing to evolve (see the $20k per month product
just launched by OpenAI) —and the best models will balance customer adoption, revenue growth, and cost predictability.
Final Thoughts
AI pricing isn’t just a cost-recovery exercise—it’s a key driver of product adoption and long-term success.
The best AI pricing models will:
✅
Align costs with value delivered.
✅
Encourage broad adoption without scaring off customers.
✅
Balance revenue predictability with scalability.
No one has cracked the perfect model yet. But over the next 12 months, we’ll learn a lot from the companies leading the way.
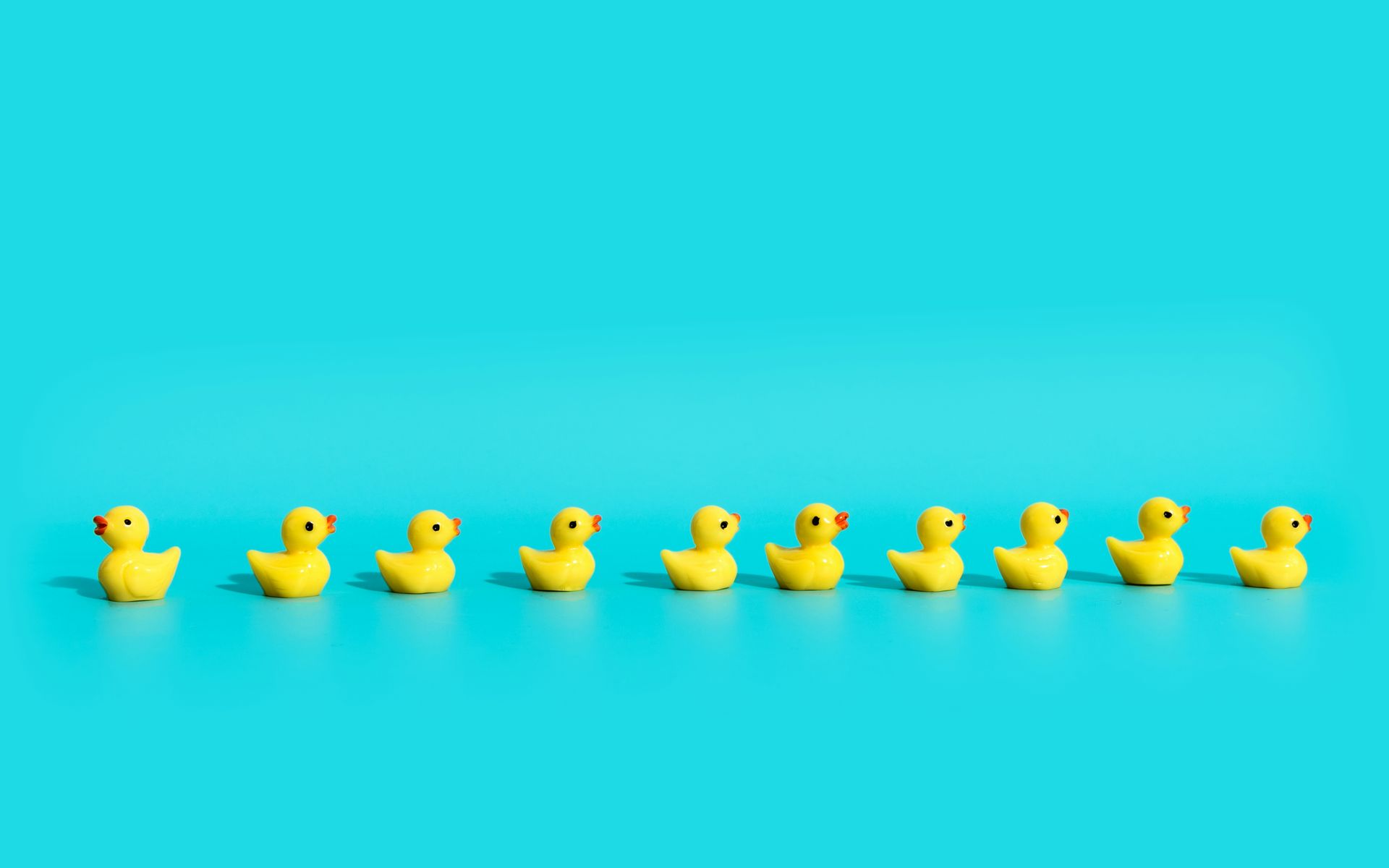